
- FORACASTING USING HIDDEN MARKOV MODEL MATLAB DRIVERS
- FORACASTING USING HIDDEN MARKOV MODEL MATLAB UPDATE
- FORACASTING USING HIDDEN MARKOV MODEL MATLAB DRIVER
- FORACASTING USING HIDDEN MARKOV MODEL MATLAB SOFTWARE
FORACASTING USING HIDDEN MARKOV MODEL MATLAB DRIVER
When a driver takes a new route, the model for this driver could not predict the driver’s route and destination intent. However, in fact, it is impractical to build a Hidden Markov Model for every driver, and many routes are not fully regular. So based on this underlying premise, they presented an approach to predict driver intent using Hidden Markov Models.

FORACASTING USING HIDDEN MARKOV MODEL MATLAB DRIVERS
firstly assumed that drivers have certain routine routes and that, by learning a model based on previous experience, one can accurately predict what a driver will do in the future. So based on this fact, they predicted a driver’s entire route by using drivers’ trip history. Froehlich and Krumm found that a large portion of a typical driver’s trips are repeated from the collected GPS data. Our method makes long-term predictions about the entire route. Our approach also differentiates from the short-term route prediction in Krumm’s work. In our work, the destination never needs to be inputted into the system beforehand. Karbassi and Barth described a method to predict smart vehicles’ routes between given starting and ending drop-off stations based on a car-sharing application.
FORACASTING USING HIDDEN MARKOV MODEL MATLAB UPDATE
The system will also update traffic information in real time in the near future and inform the driver to adjust the driving route as the trip progresses.Īt present, several methods of route prediction have been suggested, but there remain some problems. The method can predict which road segments are congested or smooth through route predictions.
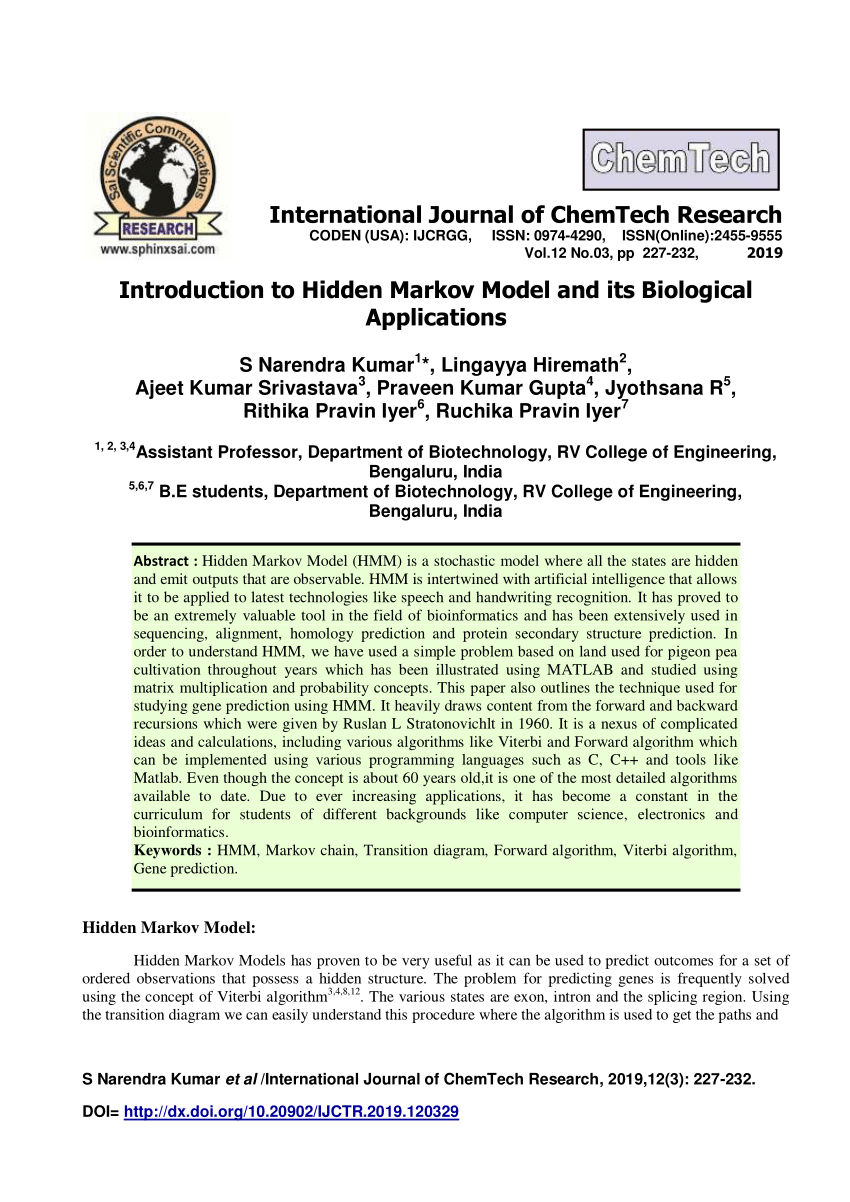
In view of the above problems, a driving route recommendation system is proposed and highlights a method for driving route predictions based on the knowledge of Hidden Markov Model (HMM). Obviously, this case may not have happened in previous historical data. While some emergency situations arise, for example, when organizing a large rally in an area, a large number of vehicles will move to this region in a short time, leading to traffic congestion in the area.
FORACASTING USING HIDDEN MARKOV MODEL MATLAB SOFTWARE
Finally, most of traffic route navigation software programs rely on historical data to predict traffic congestion. Secondly, once a route is selected, the software could not timely inform the driver to adjust the route according to real-time traffic congestion data as the trip progresses. Thus, the original relatively smooth roads will become congested and the original congested roads will become smooth. Firstly, more people would like to choose routes with many smooth road segments. However, the above methods for driving route recommendation have some problems. People usually would like to choose routes with more smooth roads.

And then a driver could select one of those recommendation routes according to personal preference, driving distance, and current road congestion information. The main function of vehicle route recommendation in the software is to find several routes between given origins and destinations by combing some path algorithms with historical traffic data, for example, Google Map and Baidu Map. IntroductionĬurrently, many drivers use different kinds of navigation software to acquire better driving routes. Finally, the experimental results of the effectiveness of the route predictions that is based on HMM are shown.

Thirdly, we present the route prediction algorithm. Secondly, we define a road network model, normalize each of driving routes in the rectangular coordinate system, and build the HMM to make preparation for route predictions using a method of training set extension based on K-means++ and the add-one (Laplace) smoothing technique. Firstly, we propose the route recommendation system architecture, where route predictions play important role in the system. This method can accurately predict a vehicle’s entire route as early in a trip’s lifetime as possible without inputting origins and destinations beforehand. We present a driving route prediction method that is based on Hidden Markov Model (HMM).
